Business Leader’s Guide to the Implementation of GenAI - Opportunities and Shortfalls
Discover how business leaders can effectively implement Generative AI in their organizations. Explore the opportunities it brings and the potential shortfalls to watch out for in our comprehensive guide.
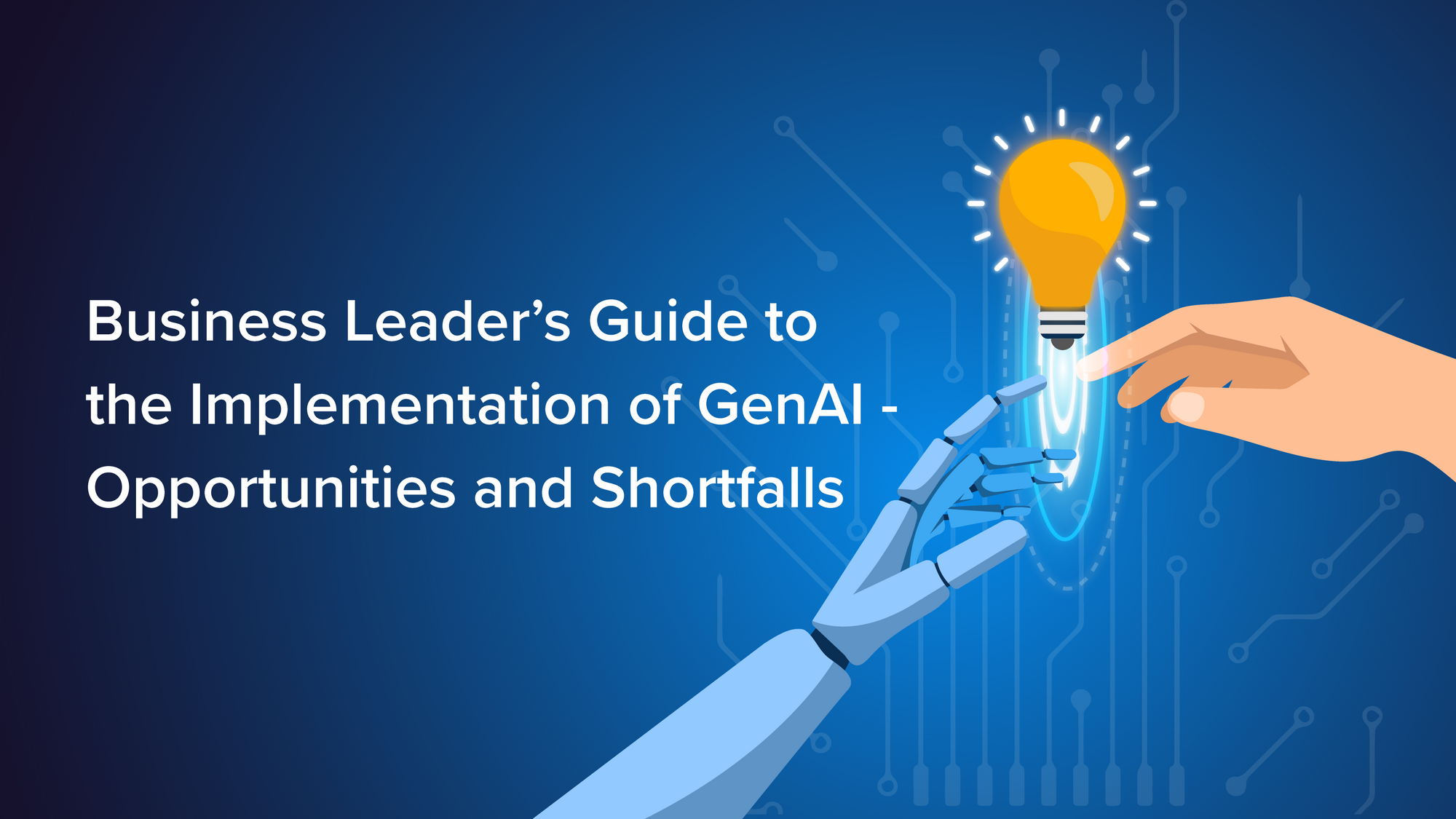
In our previous deep dive of Generative Artificial Intelligence (GenAI), we understood its genesis, objectivities with which it was conceptualised and realised as a definitive product of utility, and broad categories of GenAI products and service available to the masses. But, real world applicability is often tried and tested in the enterprise world, where value creation should far exceed resource utilization. Or in simpler terms, the value of a product of innovation should surpass the amount resources consumed in developing said product. Only then, procuring/purchasing a “product of innovation” make sense to a business leader, who looks for the real world value of such innovations.
Business Leaders’ take on the initial products released around GenAI was quite similar. Most of them, started asking the question, “How does GenAI make sense to me or my business? What kind of value does it bring?” And they were absolutely right to do so.
Some of the first products released by firms such as OpenAI—GPT 2—were nothing more than a showcase of what GenAI is capable of producing as an output. An interactive chatbot that answered questions better and with more contextual awareness than Google or Bing or Siri. But GPT-4, Claude, and Gemini, in their 3rd or 4th commerical releases have shown substantive evidence of real world use cases, where the Large Language Models (LLMs) could be trained to address definitive pain points.
Understanding the Scope
Before we delve into specific industries and GenAI applications which address such use cases, it's crucial to define a guide that focuses on specific industries, the type GenAI use cases solving a well-defined problem, implementation practices, the potential shortfalls and opportunities to look into.
- Industries: In this deep dive, let's look at retail, finance, healthcare, manufacturing, and others.
- GenAI Types: Based on its underlying architecture, the following types of GenAI frameworks could be considered for this guide: Primarily Generative Adversarial Networks (GANs), Variational Autoencoders (VAEs), and Large Language Models (LLMs).
- Implementation Steps: Define a general framework outlining key stages of implementation, and the best practices that should be ideally followed.
- Shortfalls and Opportunities: Potential challenges and benefits to be reaped in the overall Lifecyle of implementation and operational time period.
- Customer Value: How GenAI can enhance customer experiences.
Industries Mapped Across Applicability of GenAI
When popular surveys of GenAI applicability are looked at, the derived findings paint an interesting picture. And such information can be used to view historical course of events to hypothesise possible outcomes in the future while building a guide for best practices to be followed in the implementation of GenAI . The data below showcases some of the most popular real-world use cases being validated across the world using GenAI.
Industry | GenAI Type | Application | Implementation Steps | Shortfalls | Opportunities | Customer Value |
---|---|---|---|---|---|---|
Retail | GANs | Image Generation | Data Collection, Model Training, Generation, Evaluation | Lack of control over generated images, Ethical concerns | Personalized product recommendations, Virtual try-ons | Enhanced shopping experience, Increased sales |
Finance | LLMs | Fraud Detection | Data Collection, Model Training, Deployment, Monitoring | False positives, Model bias | Improved fraud prevention, Faster response times | Increased trust, Reduced losses |
Healthcare | VAEs | Drug Discovery | Data Collection, Feature Engineering, Model Training, Generation | Computational cost, Interpretability | Accelerated drug development, Personalized medicine | Improved patient outcomes, Lower costs |
Manufacturing | GANs | Quality Control | Image Data Collection, Model Training, Defect Detection | False negatives, Data quality issues | Improved product quality, Reduced defects | Increased customer satisfaction, Cost savings |
💡 Note: This table provides only a high-level overview. Each entry can be expanded into a detailed section with specific examples, case studies, and quantitative data.
Key Observation: When we look at the above table, of how GenAI could be applicable to specific use cases, we can segment the areas within which variations of GenAI could be applied:
- In Retail, gaining control over branding material created;
- In Finance, eliminating false positives and model biases;
- In healthcare, reducing computational cost involved in data collection and validation;
- In Manufacturing, improving quality control.
Conclusion: The suggested areas of applicability are conventionally overseen by unskilled labour, who may or may not possess necessary understanding of broader business objectives, presenting the opportunity for automation and up-skilling. Without adding more man power, existing resources could be trained to utilize GenAI capabilities to improve operational efficiency. In the above observations, GenAI could improve the rate at which branding materials are created for the retail sector, freeing up manpower that would be otherwise responsible, creating, refinement and publishing of said resources. Similarly, in Finance, GenAI could empower humans responsible for financial audits and anomaly detection in automating time consuming, repetitive task, such that they can free up their bandwidth to audit the created information more efficiently.
Following the same train of thought, across similar industries as noted above, GenAI can help
1) identify critical data points within a vast data sets,
2) enrich raw data into meaningful information that is easily accessibly while eliminating redundancies and false positives
3) create new, original resources based on previously gathered information in accordance with an established framework.
These three findings essentially tell us how well GenAI can help business owners optimise their revenue generating functions by eliminating redundancies in workflows while also up-skilling existing labour to drive more granular and actionable outcomes across their enterprise. Engineers such as Codemonk have improved upon these findings to address specific pain-points in the industry, serving as a testament to GenAI’s capabilities.
For better understanding, lets categorize the implementation of GenAI across the following dimensions:
- Data: The type of data required to train the model.
- GenAI Model: The most suitable type of GenAI for the application.
- Deployment: Steps involved in integrating the model into the business process.
- Maintenance: Ongoing requirements to ensure model performance.
- Operational Overheads: Costs associated with implementation and operation.
- Expected Results: Potential outcomes and benefits.
- Opportunities and Shortfalls: Advantages and challenges of the application.
Now, lets map these dimensions across industries and observe the best practices to be followed.
Category | Description |
---|---|
Data | Customer purchase history, product information, sales data, customer demographics, market trends |
GenAI Model | GANs for image generation, LLMs for customer service, recommendation systems |
Deployment | Integration into e-commerce platforms, point-of-sale systems, customer relationship management (CRM) |
Maintenance | Continuous data updates, model retraining, performance monitoring |
Operational Overheads | Data storage, computational resources, model development costs |
Expected Results | Personalized product recommendations, improved customer experience, increased sales, optimized inventory management |
Opportunities & Shortfalls | Potential for hyper-personalized marketing, risk of privacy breaches, model bias |
Healthcare
Category | Description |
---|---|
Data | Patient records, medical images, clinical data, research papers |
GenAI Model | VAEs for medical image analysis, LLMs for drug discovery, patient diagnosis |
Deployment | Integration into electronic health records (EHR), medical imaging software, research platforms |
Maintenance | Data privacy compliance, model validation, regulatory approvals |
Operational Overheads | High computational costs, data security, ethical considerations |
Expected Results | Improved diagnosis accuracy, accelerated drug discovery, personalized treatment plans |
Opportunities & Shortfalls | Potential to save lives, ethical concerns around patient data, model interpretability |
Manufacturing
Category | Description |
---|---|
Data | Production data, equipment sensor data, supply chain data, product design data |
GenAI Model | GANs for quality control, VAEs for predictive maintenance, LLMs for supply chain optimization |
Deployment | Integration into production lines, maintenance systems, supply chain management software |
Maintenance | Data cleaning, model retraining, equipment calibration |
Operational Overheads | Hardware infrastructure, data storage, model development costs |
Expected Results | Increased production efficiency, reduced downtime, improved product quality, optimized supply chain |
Opportunities & Shortfalls | Potential for cost savings, dependence on data quality, implementation challenges |
Computer Vision
Category | Description |
---|---|
Data | Images, videos, metadata |
GenAI Model | GANs for image generation, object detection, image segmentation |
Deployment | Integration into image processing software, video surveillance systems |
Maintenance | Data labeling, model accuracy evaluation, hardware upgrades |
Operational Overheads | Computational resources, data storage, software licenses |
Expected Results | Improved image quality, enhanced object recognition, automated image analysis |
Opportunities & Shortfalls | Potential for new applications, ethical concerns around image manipulation, model bias |
Finance
Category | Description |
---|---|
Data | Financial transactions, market data, customer data, risk assessment data |
GenAI Model | LLMs for fraud detection, risk assessment, financial forecasting |
Deployment | Integration into financial systems, trading platforms, customer service portals |
Maintenance | Data security, model retraining, regulatory compliance |
Operational Overheads | Data storage, computational resources, model development costs |
Expected Results | Improved fraud prevention, better risk management, personalized financial advice |
Opportunities & Shortfalls | Potential for increased profits, regulatory challenges, model explainability |
Customer Support and Service
Category | Description |
---|---|
Data | Customer inquiries, support tickets, customer feedback |
GenAI Model | LLMs for chatbots, sentiment analysis |
Deployment | Integration into customer support platforms, websites |
Maintenance | Continuous model training, performance evaluation |
Operational Overheads | Natural language processing (NLP) tools, infrastructure costs |
Expected Results | Improved customer satisfaction, reduced response times, increased efficiency |
Opportunities & Shortfalls | Potential for 24/7 support, reliance on data quality, risk of negative sentiment amplification |
Marketing
Category | Description |
---|---|
Data | Customer demographics, purchase history, market trends, social media data |
GenAI Model | GANs for image generation, LLMs for content generation, sentiment analysis |
Deployment | Integration into marketing automation platforms, social media management tools |
Maintenance | Data updates, model retraining, performance tracking |
Operational Overheads | Data collection, model development, marketing tools |
Expected Results | Personalized marketing campaigns, increased engagement, improved brand reputation |
Opportunities & Shortfalls | Potential for increased sales, ethical concerns around data privacy, model bias |
Although the above best practices tell us what the expected outcome could be when GenAI is applied across the above industries, a few points to be considered during GenAI explorations are noted below. These considerations are intended to avoid any pitfalls a business owner might stumbled upon while also keeping an eye out for opportunities for innovation.
Key Considerations
- Data Quality: While building said GenAI models, business owners have to pay close attention to the Accurate and representative data to ensure optimal model performance. How such data is collected, managed, and used plays a vital role in the end outcome achieved.
- Model Selection: The many variations of GenAI, as presented above, showcase distinct capabilities. And as such, the selection of GenAI models, particularly training algorithms, has to be done meticulously, wherein the model matures as per the training data to produce expected outcomes. Businesses need to choose the appropriate GenAI model based on the specific use case.
- Ethical Implications: Consider the ethical implications of using GenAI, such as bias and privacy. Based on the data with which an LLM is trained, GenAI could showcase implicit bias in produce results. To address the same, businesses need to further train and refine the LLMs with relevant historical information.
- Continuous Improvement: Regularly update and refine models to maintain performance. With the passing of each data, GenAI models have to be trained successively with new information to align it as per organisational objectives.
- Talent Acquisition: Build a team with AI expertise to drive successful implementation. Though the output of GenAI solutions produce accurate results based on training data, refining the same for much more granular insights. Essentially, TAs will be up-skilling the workforce tasked for GenAI optimization.
By carefully considering these factors, businesses can effectively leverage GenAI to drive innovation and improve operational efficiency.