Codemonk Skout - Video Intelligence for Football Analytics
Our video intelligence capabilities are finetuned in accordance with the footballing world, analyzing video feeds frame by frame, identifying objects, people, scenes, and other contextual elements to provide valuable insights to various stakeholders in the sporting value chain
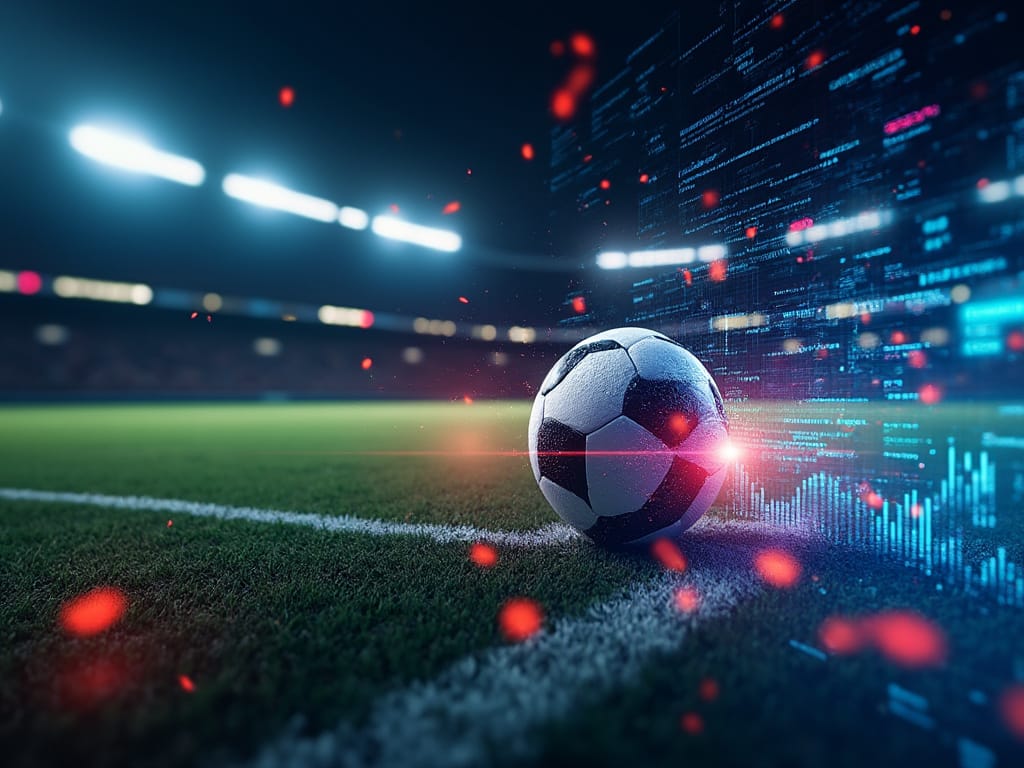
When your profession intersects with a hobby or a genuine area of interest, you find yourself working on a product that not only interests you as a prospective consumer, but also opens promising avenues of applicability across a wider community that finds equivalent value in the product. Thats what happened when I started working on Skout, with the tech team at Codemonk.
Previously, we had spoken at great lengths about our video intelligence capabilities, using which we were able to generate life-like commentary on sporting events, some of which were captured using smartphone cameras. We tasked our video intelligence tool to create life-like commentary on Gully Cricket artificially voiced by legends such as Ravi Shashtri, for video footage recorded by people using rudimentary camera equipment from a small township in india. Within no time, we received enquiries for Live Commentary on Sporting events taking place in the local provinces of the Republic of Ireland. Again, within no time, we fine tuned our video intelligence capabilities in accordance with the footballing world, analyzing video feeds frame by frame, identifying objects, people, scenes, and other contextual elements to provide valuable insights to various stakeholders in the sporting value chain.
With the fruition of Skout, we were able to validate 10 specific use cases within the sporting realm. Here are the findings as per applicability validated. Although the solution is still running on terminal, we are able to produce noteworthy outputs. Below, we detail each use case validated by Skout as of 7th October 2024.
1. Real-time Performance Analytics
Use Case
Provide coaches and analysts with instant, detailed performance metrics during live matches.
Problem Statement
Coaches need real-time data to make informed tactical decisions during matches, but traditional methods of data collection and analysis are often too slow or limited in scope.
Data Feed (Input) Available
Live match footage from multiple camera angles.
Type of Analytics Performed
- Player movement tracking
- Ball possession analysis
- Formation recognition
- Individual player action recognition (passes, shots, tackles, etc.)
Type of Insights Derived
- Heat maps of player positioning
- Pass completion rates and patterns
- Team formation changes over time
- Individual player performance metrics
Example
During a Premier League match between Manchester City and Liverpool, the video intelligence solution is applied to map players and assets on the football field (such as the ball and the goalposts, corner flags and more). The movement and changes made to the position of any of these elements is recorded in real time. For example, during the Line-up, each player’s jersey number is marked down as a unique identifier, that allows multiple cameras placed on field to recognise the player at any instance in time.
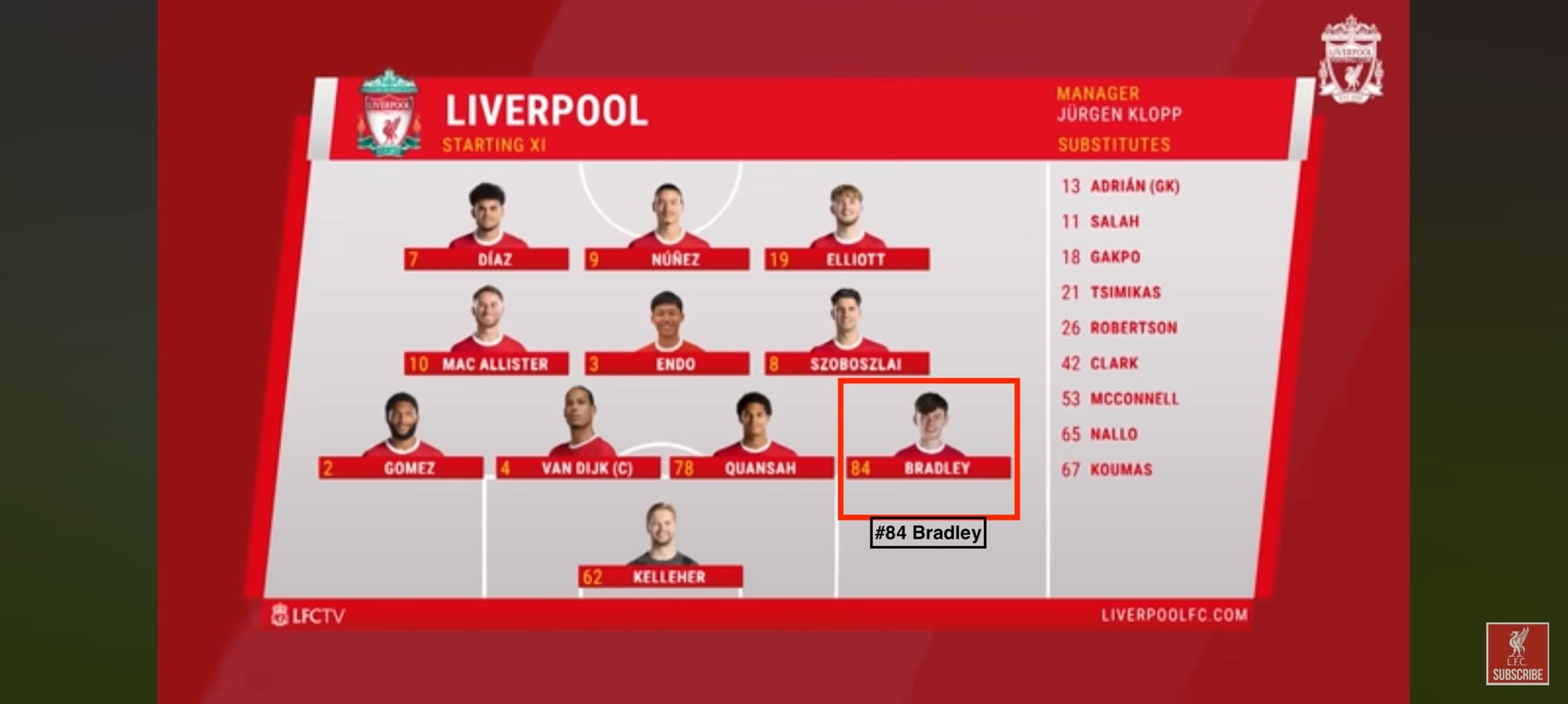
Next, during the match, the same technology tracks Kevin De Bruyne's (the player under observation) movements and actions. It identifies that he's completing 95% of his passes in the final third and has created 5 high-quality chances in the first half. The coach uses this information to adjust tactics, instructing other players to feed De Bruyne more often in attacking positions.
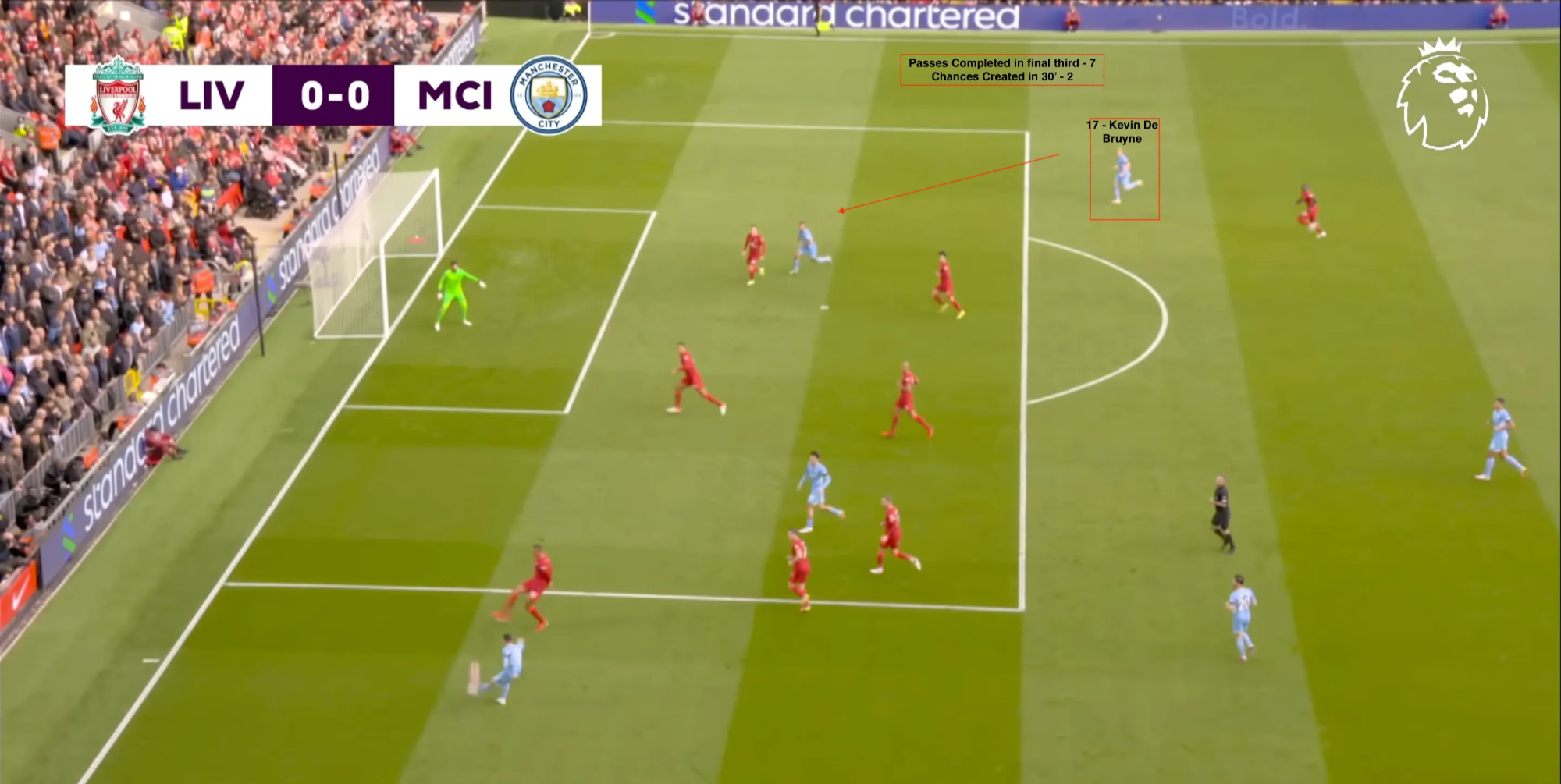
2. Automated Highlight Generation
Use Case
Create instant, customized highlight reels for broadcasters and social media teams.
Problem Statement
Producing engaging highlight packages quickly after matches is labor-intensive and can miss key moments. The below example shows a chance created by Liverpool, just narrowly beating the keeper and the goalpost. The shot is deemed as “off target” for missing the post, while the trajectory of the ball and the speed of the ball is tracked in real time. Although this is a missed opportunity for the striker attempting the shot, these metrics allow coaches to understand how many chances are getting created and converted on field based on the applied tactical formation and strategy.
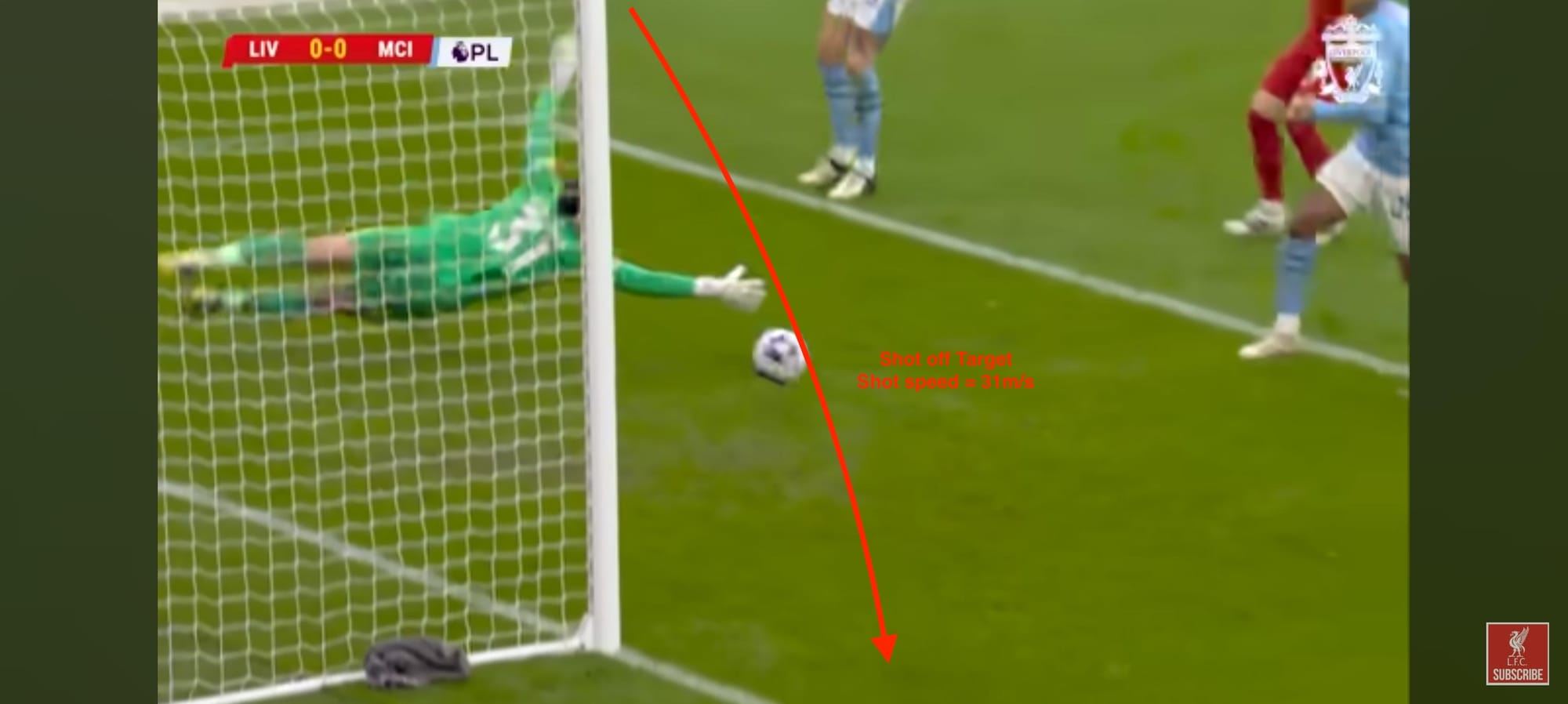
Data Feed (Input) Available
Full match recordings, including multiple camera angles and replay footage.
Type of Analytics Performed
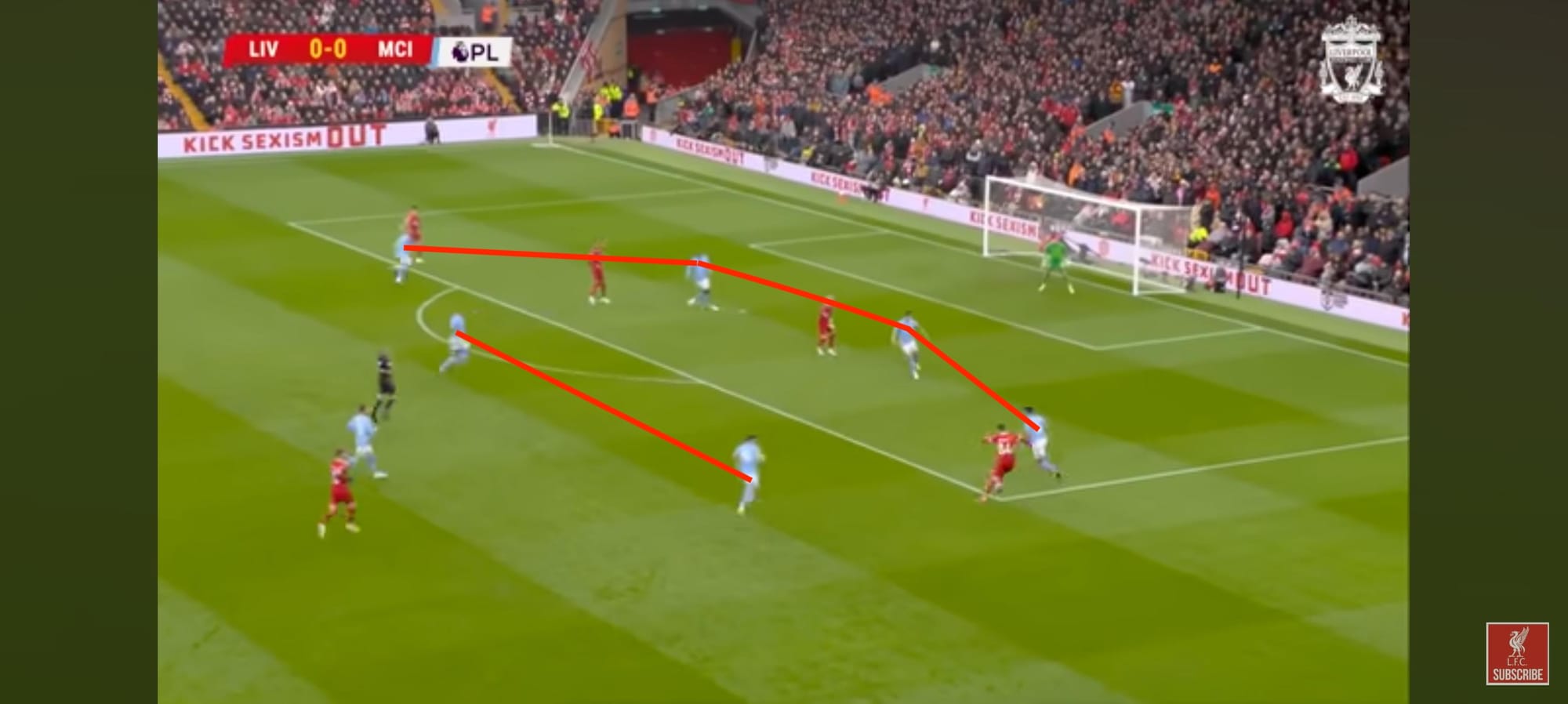
Here, we can see the tactical formation taken up by Manchester City when defending a counterattack, allowing us to index information such as,
- Key event detection (goals, near misses, saves, fouls, etc.)
- Crowd reaction analysis
- Player emotion recognition
- Commentary audio analysis
Type of Insights Derived
- Automated tagging of significant moments
- Excitement level scoring for each event
- Player celebration and reaction clips
- Commentary peak identification
3. Referee Decision Analysis
Use Case
Assist match officials and VAR (Video Assistant Referee) teams in making accurate decisions.
Problem Statement
Controversial decisions can significantly impact match outcomes, and current VAR processes can be time-consuming and still prone to human error. In the below example, Liverpool keeper commits a foul on the city forward, inside the D-box, without gaining possession of the ball. Here, this real time insight would be given to the VAR, thereby ensuring that correct decisions are made on the pitch, through the recognition of fouls committed by players according to the rulebook.
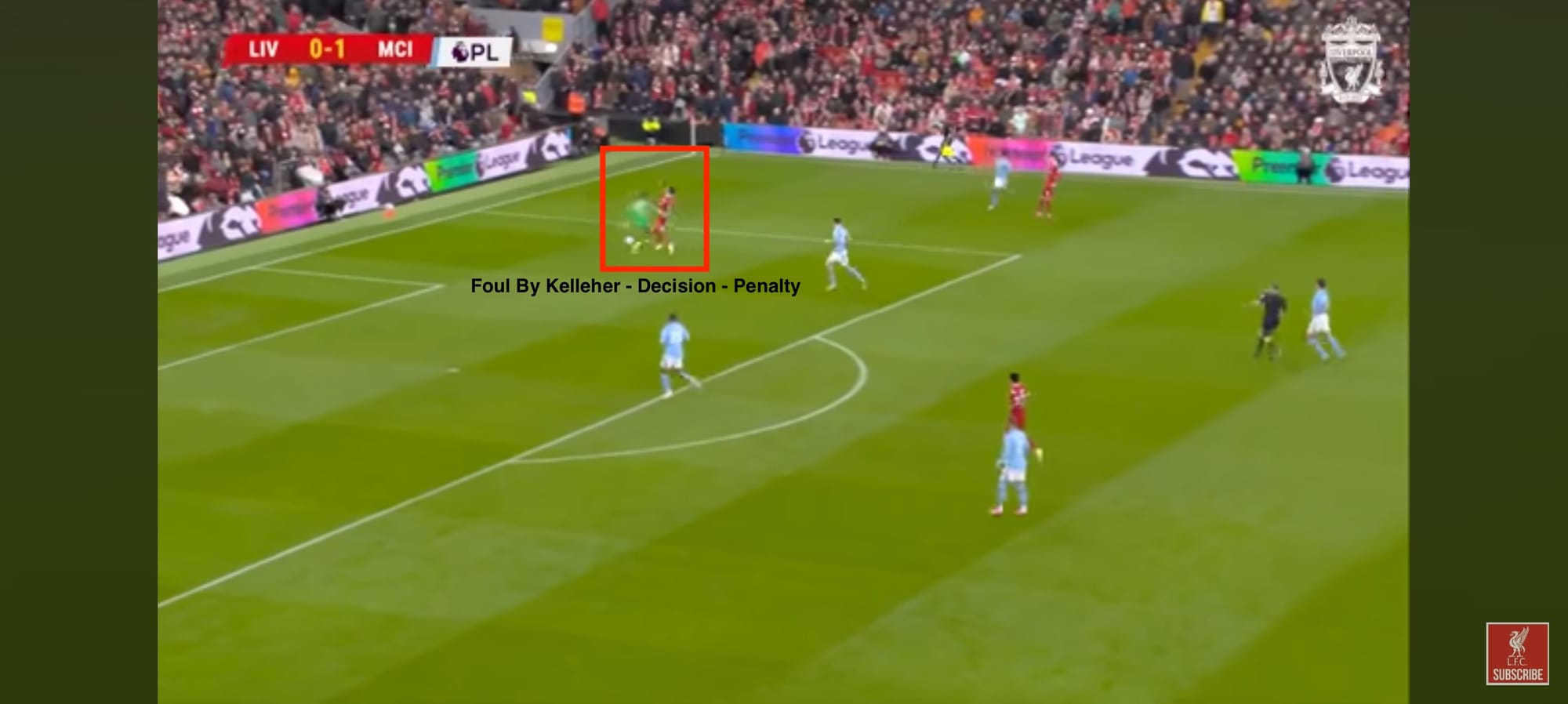
Data Feed (Input) Available
Multi-angle match footage, including high-frame-rate cameras.
Type of Analytics Performed
- Offside line detection and player positioning
- Ball trajectory and goal-line analysis
- Player contact detection for foul assessment
- Handball detection
Type of Insights Derived
- Precise offside calculations with confidence levels
- Frame-by-frame analysis of potential fouls
- 3D reconstructions of controversial incidents
4. Fan Engagement and Personalized Content
Use Case
Deliver tailored content and interactive experiences to fans based on their preferences and viewing history. When connected with a broadcasting partner, on-screen information can be parsed intelligently for post match summary and highlight reel generation, fostering greater fan engagement.
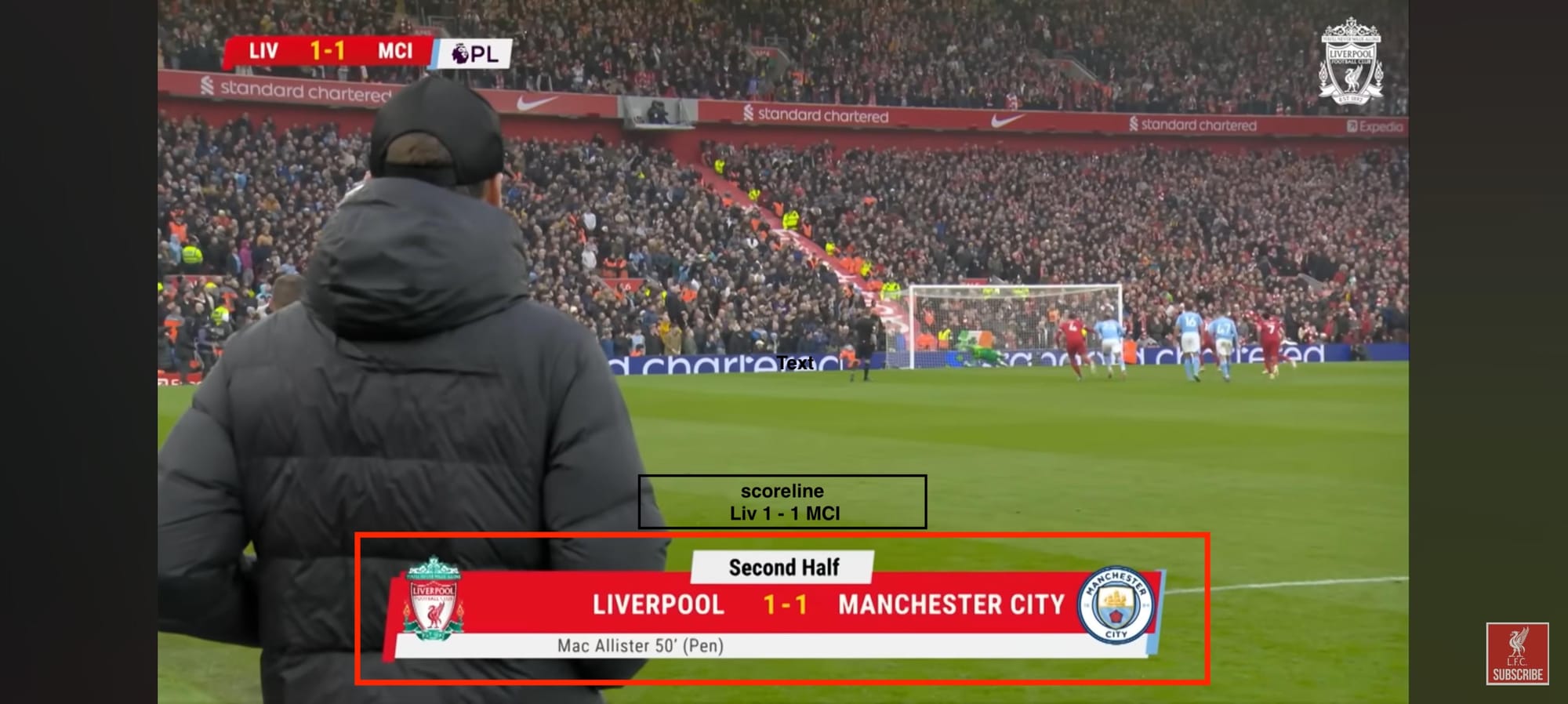
Problem Statement
Fans are overwhelmed with generic content and seek more personalized, engaging experiences that cater to their specific interests.
Data Feed (Input) Available
Live and archived match footage, user viewing history, social media interactions.
Type of Analytics Performed
- Player and team recognition
- Action classification (goals, skills, celebrations)
- Fan reaction analysis from social media feeds
- User preference modeling
Type of Insights Derived
- Personalized highlight reels
- Player-specific skill compilations
- Tailored match statistics and visualizations
- Interactive quizzes and challenges based on recent matches
5. Tactical Opposition Analysis
Use Case
Provide coaching staff with comprehensive insights into opponents' strategies and player tendencies.
Problem Statement
Preparing for opponents requires extensive manual video analysis, which is time-consuming and may miss subtle patterns. As clearly evident in the below shot, the city player is left unmarked when the ball is crossed into the penalty box from the corner. Insight allows Liverpool coaching staff to reorganise their defensive strategy to better mark players would could connect with the cross delivered in the corner taken.
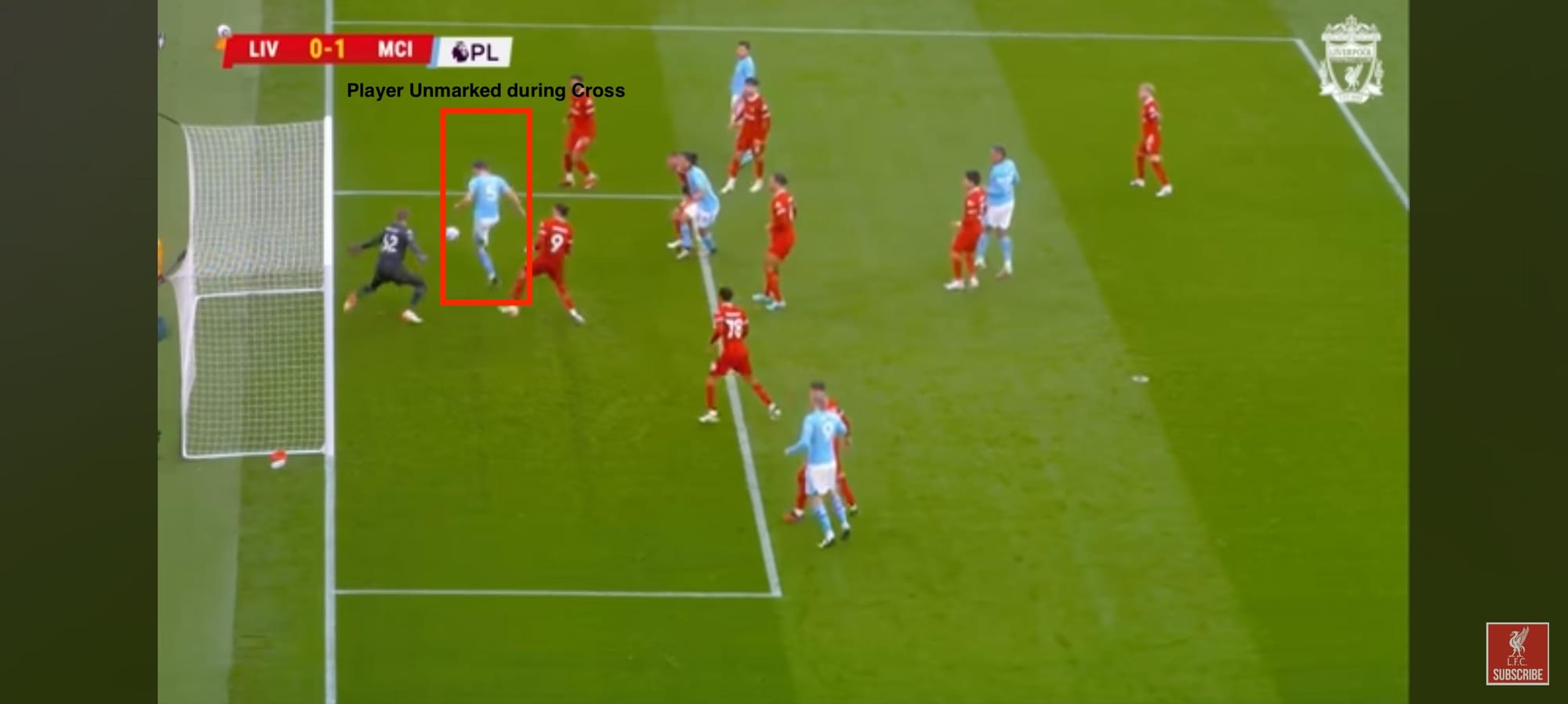
Data Feed (Input) Available
Historical match footage of upcoming opponents, training session videos.
Type of Analytics Performed
- Team formation and tactic recognition
- Individual player tendency analysis
- Set-piece pattern identification
- Pressing and defensive structure analysis
Type of Insights Derived
- Heatmaps of opponent formations in different game scenarios
- Predictive models of player movements and decisions
- Weakness identification in defensive structures
- Customized reports on opponent tendencies under pressure
6. Injury Prevention and Player Wellness
Use Case
Monitor player movements and exertion levels to predict and prevent potential injuries.
Problem Statement
Player injuries can significantly impact team performance and club finances, but current methods of predicting and preventing injuries are limited.
Data Feed (Input) Available
Training session footage, match recordings, historical injury data. Such actionable information is gathered continuously for post training analysis and pre-training preparation. Below, in a game of shuttle, Team-Blue shuttles the ball between players which Team-Orange attempts to gain possession of the ball. The response time for each players reacting to a pass made is recorded in real time, showcasing the agility of each player on pitch and when passed the ball.
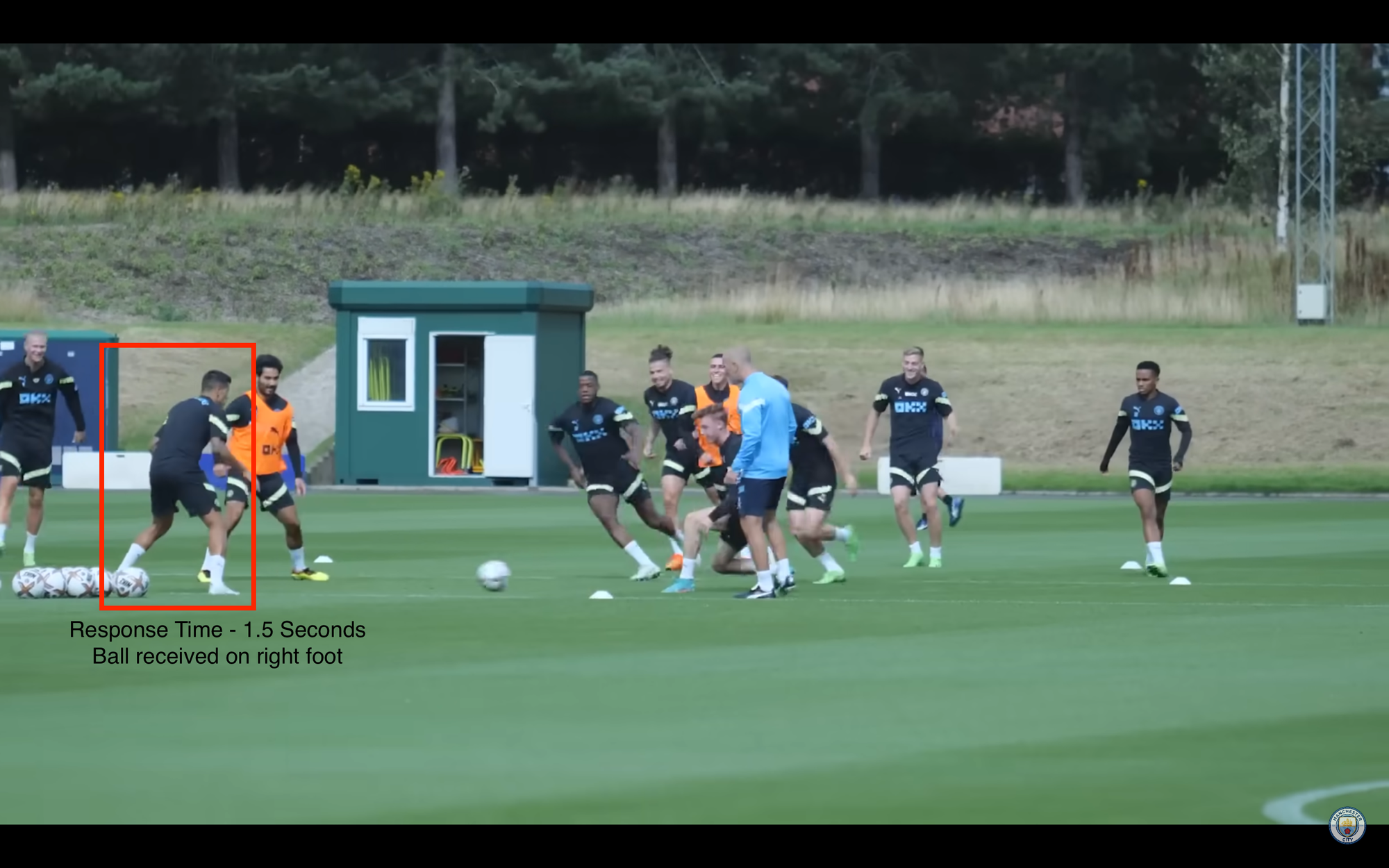
Type of Analytics Performed
- Biomechanical analysis of player movements
- Fatigue indicator recognition
- Comparison of movement patterns with historical injury data
- Playing time and intensity tracking
Type of Insights Derived
- Early warning signs of potential injuries
- Personalized training load recommendations
- Risk assessments for different playing styles and positions
- Long-term player development and injury prevention strategies
7. Scouting and Player Recruitment
Use Case
Enhance the scouting process by providing comprehensive player analysis across multiple leagues and levels. Players can be monitored at a grassroots level using on field handheld devices for continuous training development and progression tracking.
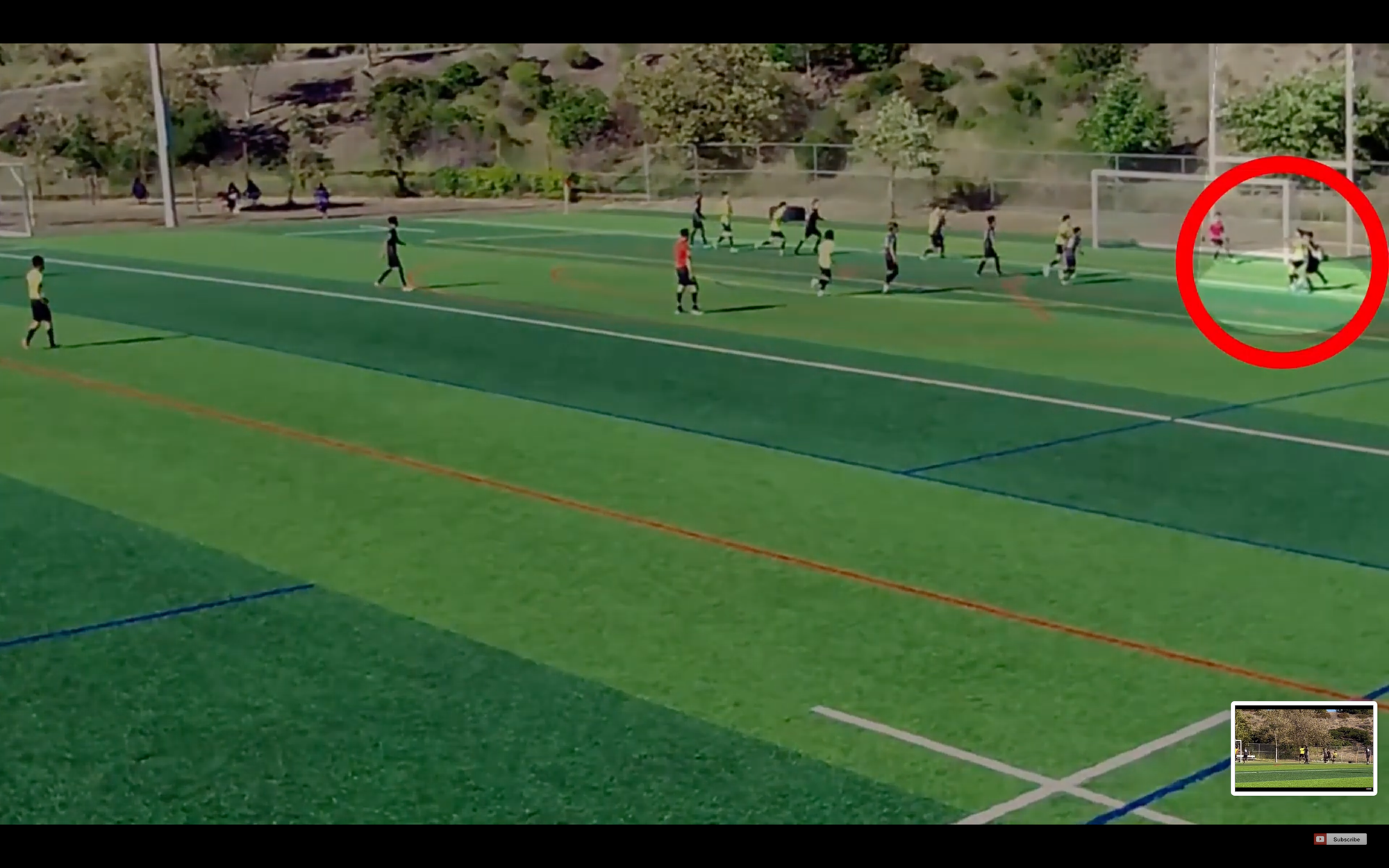
Problem Statement
Traditional scouting methods are resource-intensive and can miss talented players in less-covered leagues or lower divisions.
Data Feed (Input) Available
Match footage from various leagues, including lower divisions and youth competitions.
Type of Analytics Performed
- Player skill assessment (passing, shooting, dribbling, etc.)
- Physical attribute analysis (speed, stamina, strength)
- Tactical awareness evaluation
- Performance consistency tracking
Type of Insights Derived
- Comparative player rankings across different leagues
- Potential transfer target identification
- Player development trajectory predictions
- Compatibility assessments with team playing styles
Using these insights various participants of the football value chain can proactively take decisions that improve the team as whole. Codemonk is actively engaging with partners to collect more data points that enable coaches and managers to measure progress on a regular basis.
We also invite development community to contribute in any fashion they deem fit towards the platform, if they choose to, as we try make the platform more accessible to all.
Reach out to us at:https://codemonk.io/contact