The story of GenAI - An Explorer of Fundamental Concepts
Explore the fundamentals of Generative AI with our comprehensive guide, 'The Story of GenAI - An Explorer of Fundamental Concepts.' Discover the technology and its applications.
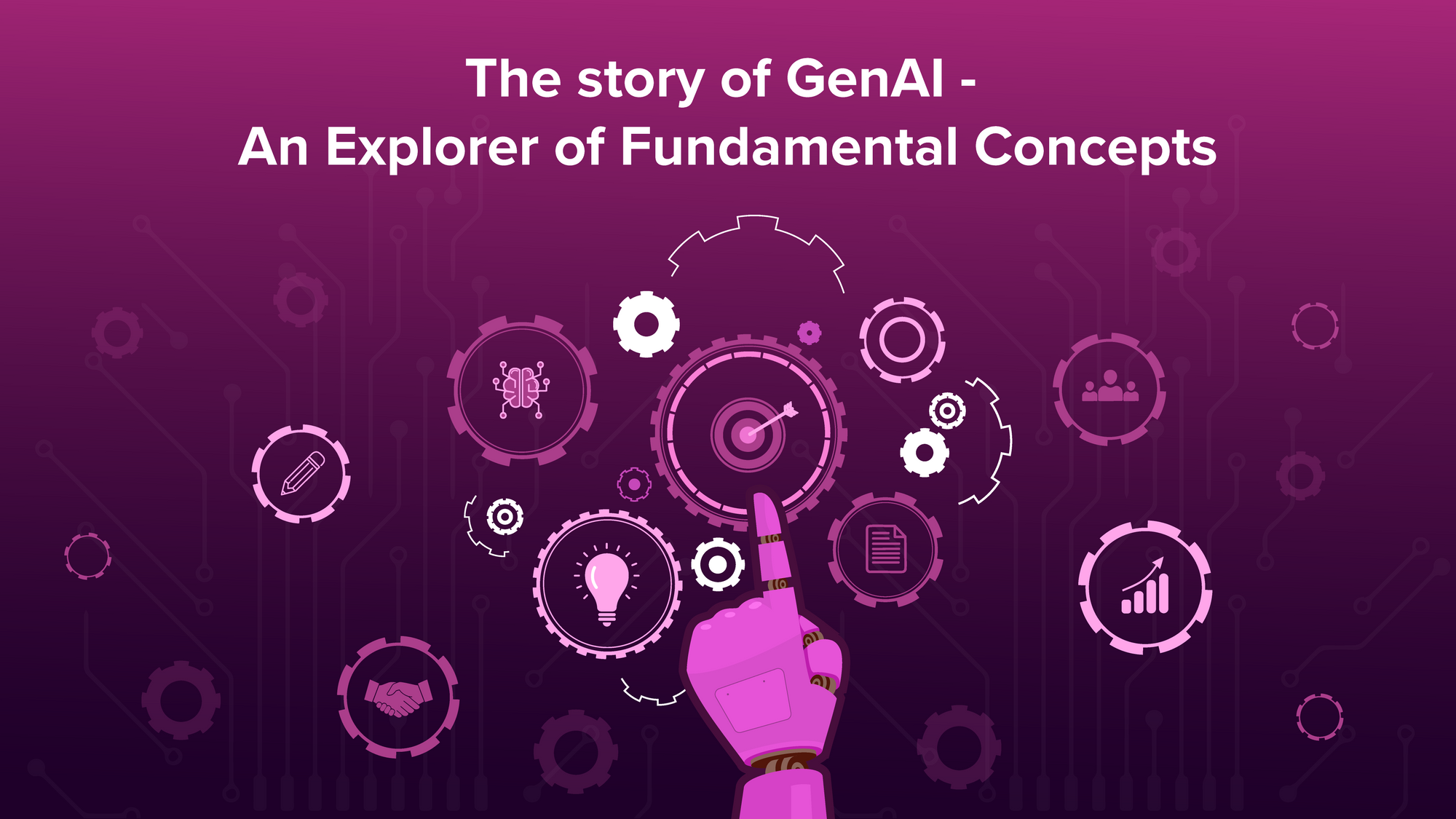
In the 1950s, when machine learning algorithms were being conceptualized, Russian mathematician Andrey Markov’s Markov chains became the talk of the town. He hypothesized in 1906 through his paper that a definite sequence of possible events can be identified using the state attained by previous events leading up to it, or in simpler words, “What happens next depends only on the state of affairs now.” When scientific exploration around machine learning algorithms was conducted by researchers worldwide, they honored Andrey’s work on identifying the occurrence probability of a sequence of events based on the historical path taken by previous events. Scientists who based their research on Andrey’s principle of identifying sequences of events were able to generate new sequences of data using existing information successfully.
And thus, machine learning algorithms were born, validating Andrey’s work. Today, these algorithms (or in layman’s terms, mathematical models) form the basis on which generative intelligence is modeled. Data scientists of the modern day develop machine learning algorithms, trained on a predefined data set to produce a definitive outcome. And, in the case of Generative artificial intelligence (GenAI), algorithms are trained on data sets to create information that bears resemblance to the data it was trained on.
For instance, if a set of GenAI algorithms is trained on a specific genre of music or artist’s composition, it would essentially be capable of creating music using the patterns identified on the previously trained data sets. Although not considered purely original, the output of these generative intelligence models differs just enough to bypass/circumvent copyright and plagiarism checks. Further refinement through human intervention produces fairly original content, which could be copyrighted as a new asset on its own. Invariably, such GenAI capabilities possess endless possibilities in enhancing the productivity of content creators, who would otherwise need to manually identify patterns, as an algorithm would, to create new, original content. Referencing the above example in the musical industry, a new musician would have to try out multiple composition styles within a selected genre to produce new music. If the same workflow is transferred onto a GenAI model, algorithms would take the onus of creating new music, which is then refined by the same musician for originality.
History and exemplars aside, what is GenAI? How is it useful to a modern-day business owner? Let's try to define GenAI across 5 levels of comprehension, understandable by
- A 10-year-old child,
- An 18-year-old student of science and technology,
- 35-year-old researcher embarking on his GenAI journey,
- An expert actively working on GenAI, and finally,
- A developer modeling his/her own algorithms.
- 10-Year-Old: Imagine you have a super cool toy box that can be broken apart into smaller building blocks, like Legos. You can build anything you want with them - cars, spaceships, even dragons! GenAI is like a magical box filled with a vast number of tiny digital Legos. You can speak to the box, and ask it to create new toys that you did not have before, all using smaller pieces/building blocks of digital legos.
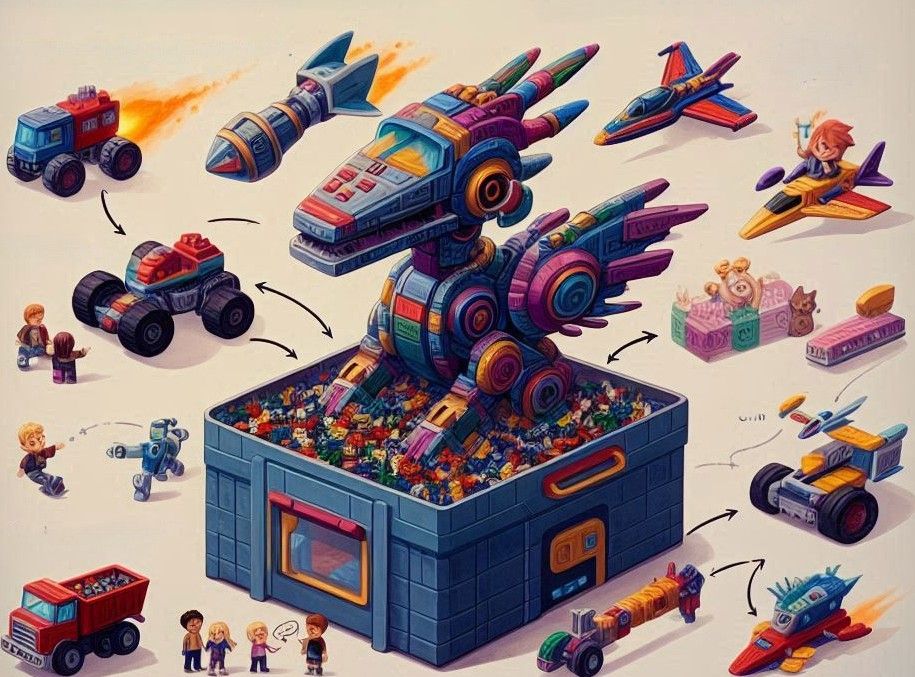
- 18-Year-Old: GenAI, abbreviated as Generative Artificial Intelligence, is a branch of AI that creates new, meaningful information. Think of it as an intelligent photocopy machine that can not only copy printed information but also use the gathered knowledge to draft or create new information on paper by identifying patterns of information used while scanning documents. It could be used for creating realistic images, writing different kinds of creative text formats, and even composing music.
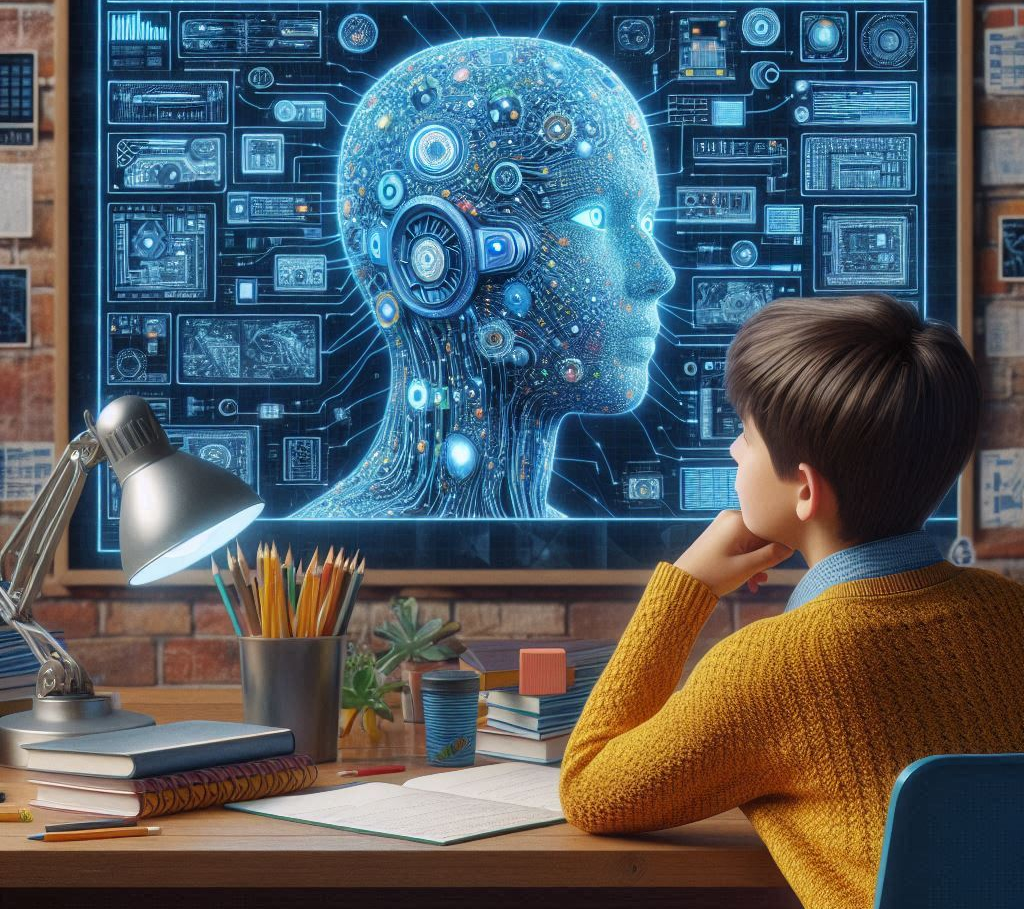
- 35-Year-Old beginning their GenAI journey: GenAI utilizes machine learning algorithms, particularly deep learning techniques like neural networks, to analyze vast amounts of existing data. This analysis allows it to learn patterns and relationships within the data. It then leverages this knowledge to generate entirely new data, mimicking the styles and formats it has learned. Common applications include Generative Adversarial Networks (GANs) which pit two neural networks against each other, one creating new data and the other trying to distinguish it from real data, ultimately leading to highly realistic outputs.
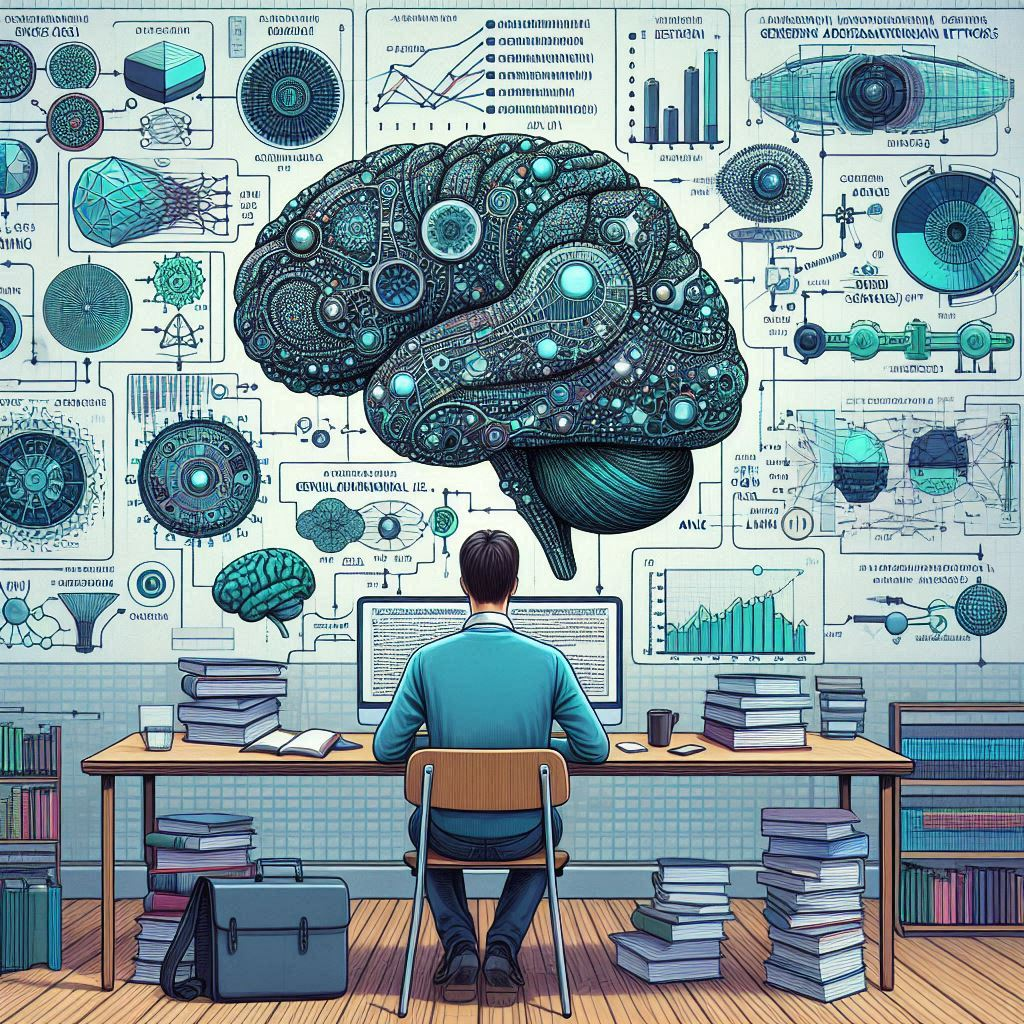
- AI Expert using: Generative AI encompasses a range of techniques for creating novel data. From Variational Autoencoders (VAEs) for efficient data representation to reinforcement learning approaches for exploring creative spaces, GenAI pushes the boundaries of machine creativity. Its impact is felt in Natural Language Processing (NLP) for text generation and translation, computer vision for image and video manipulation, and various other domains. Challenges include ensuring interpretability and mitigating potential biases within the generated data.
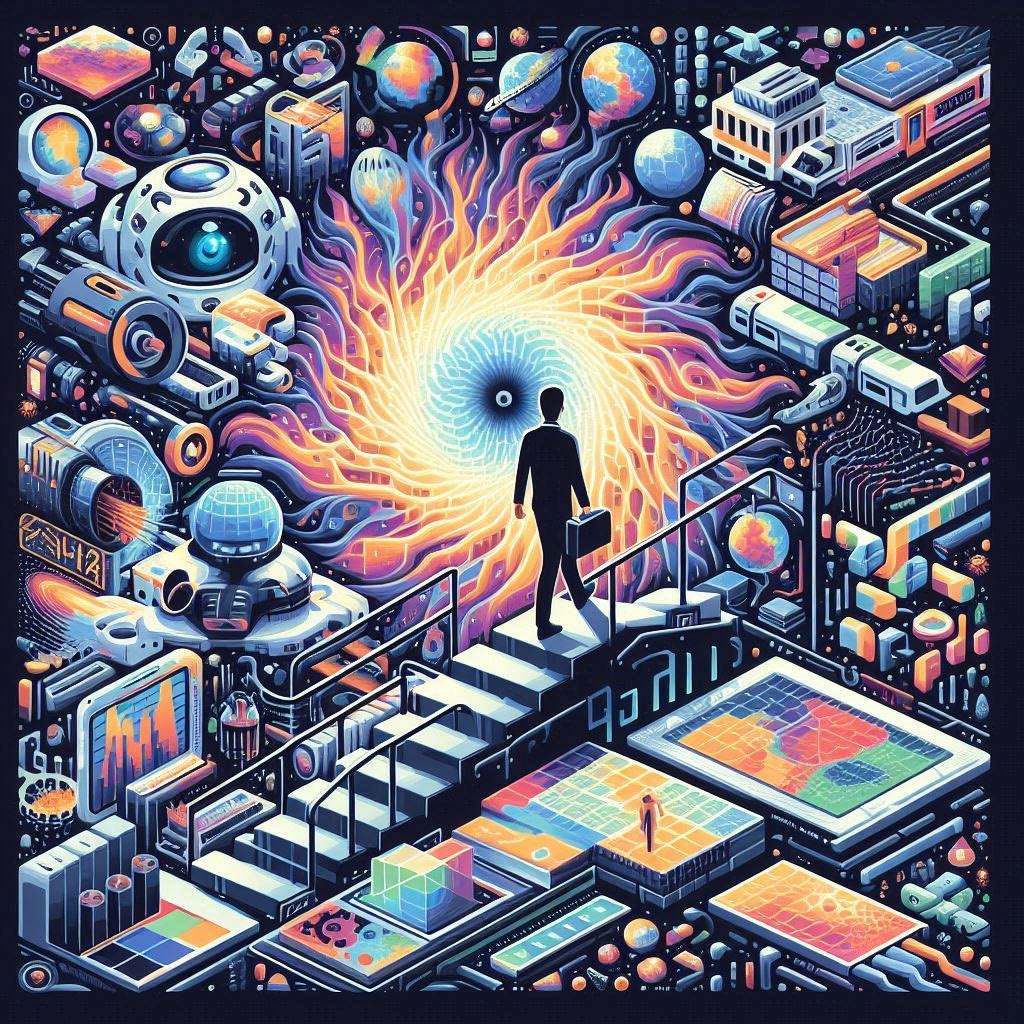
- GenAI Developer: Although preaching to the choir, consider working with the most innovative AI techniques to bring generative models to life, optimizing architectures, exploring new loss functions and training methodologies to push the capabilities of GANs, VAEs, and other generative models. Collectively, the focus lies on the efficiency, controllability, and robustness of these models to create ever-more impressive and impactful applications across various fields.
Now that definitions are out of the way, the applicability of GenAI is what entices business owners, technologists, and problem solvers for its ability to help accelerate growth by automating the generation of content across definite revenue generation pipelines. Such enthusiasts could find themselves at the crossroads of decision-making when it comes to choosing the right type of GenAI model for their business use cases. Below is a brief, yet informative classification of the types of GenAI models in existence and in development.
1) Generative Adversarial Networks (GANs): Modelled with two neural networks that compete with each other, a generator that creates new data and a discriminator that tries to identify real data from the generated data, GANs help produce original, usable content, wherein the originality of content depends on the quality of data with which the GANs are trained.
2) Variational Autoencoders (VAEs): These models recreate a compressed representation of the data (latent space); for example, an image that is compressed to a latent representation in the encoding stage, and then decoded to reconstruct the original data or generate new variations based on the latent space.
3) Autoregressive Models: These models, similar to Markov chains, generate new information in an iterative fashion by predicting the data set in a sequence of information. For example, autoregressive models can predict the next word in a sentence or the next pixel in an image using previous data inputs. This approach can be computationally expensive but can produce high-quality outputs.
4) Less popular but equally resourceful Techniques: Newer variations of GenAI models include diffusion models and flow-based models, each with their own strengths and weaknesses for different generative tasks.
We, at Codemonk, have validated numerous use cases such as
- Producing contextual information using Video Intelligence (Video AI), whereby information is gathered from media used for input such as a video file or a repository of video files to derive actionable information. Codemonk has been in successful creating video commentary for any recorded footage of a cricket match being played out, with life-like narrations, resembling the commentary styles of cricketing legends Ravi Shastri and more
- Developing an AI-driven customer support chatbot that understands communication between a service executive and a customer. This capability alone has been adopted for service audits, sales training, and conflict resolutions, among many others.
- Building an organization-level document intelligence solution that comprehends any and all data exchanged within an org to produce actionable items for decision-makers and service personnel.
Although we have several projects of similar utility, Codemonk firmly believes in offering capabilities, rather than finished products, using which businesses could build private GPTs, among many interesting and noteworthy use cases. We invite you to come to us with problem statements and realise resolutions worthy of a full-fledged showcase.