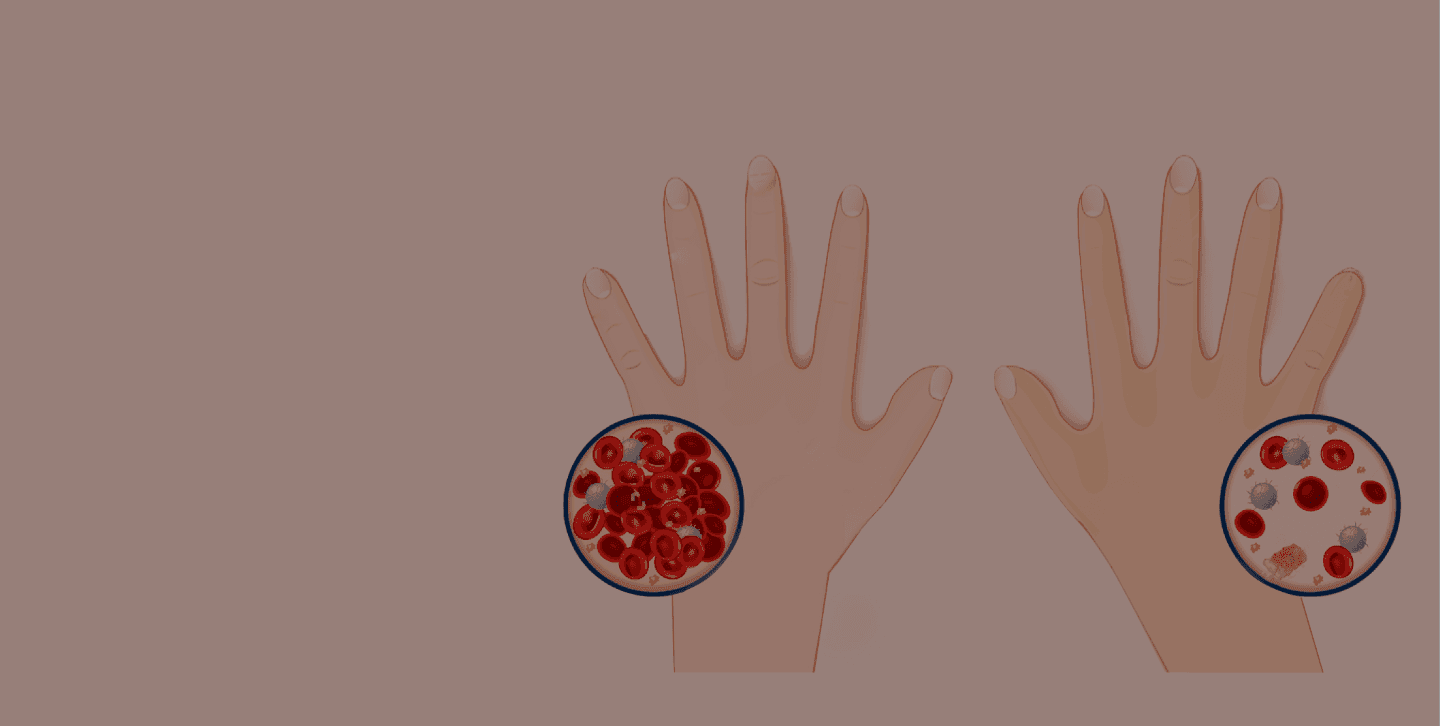
Scaling Up Anemia Detection: A Cost-Effective and Accessible Approach
Accuracy in Anemia Detection
Model Inference Time
INTRODUCTION
Anemia arises from a deficiency of healthy red blood cells, preventing the body from delivering enough oxygen to the tissues. According to the WHO, the condition is a prevalent public health problem, affecting about 2 billion people worldwide. In 2011, anemia affected 29% of non-pregnant women worldwide. Most individuals are unaware of their condition until symptoms appear. Anemia can range from temporary to long-term and is classified as Non-Anemia, Mild Anemia, Moderate Anemia, or Severe Anemia based on Hemoglobin count. While typically reversible, prompt identification and intervention are essential to reduce fatalities associated with this condition. However, current anemia detection systems fail to possess the required scalability and speed to facilitate early detection for all individuals. SDG3 Health aimed to address this challenge by developing a simple, cost-effective solution capable of widespread deployment which could impact millions of people from being affected severely due to Anemia.
Industry
HealthTech
Time Frame
1 Month
Services we offered
AI/ML, Mobile App Development
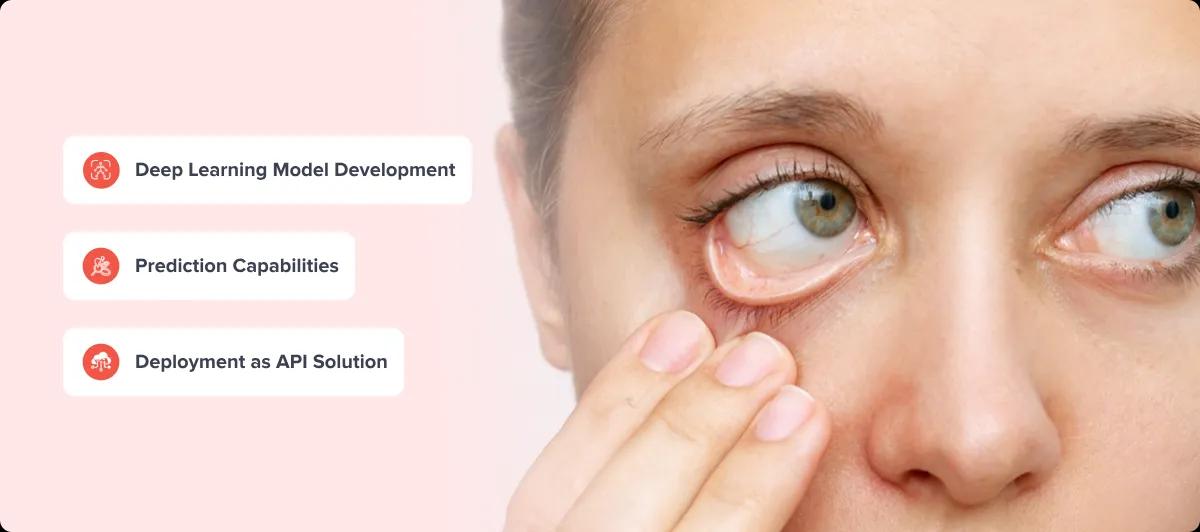
The Challenge
Existing Anemia detection systems presented three primary challenges:
Scalability
Current analysis methods rely on intricate machinery setups and skilled operators, rendering them costly, immobile, and labor-intensive. Logistical hurdles, such as sample transportation and processing, contribute to time-consuming operations and difficulty in scalability.
Invasive Approach
The majority of precise diagnostic techniques entail invasive procedures, such as blood sample collection and subsequent testing, which are inconvenient and time-consuming.
Accuracy
Although non-invasive methods exist, their precision remains a significant concern, preventing widespread adoption.
Thus, our task was to develop an anemia detection solution that prioritized scalability, non-invasiveness, and high accuracy.
Codemonk’s Approach
Following extensive research and understanding of anemia, our goal was to devise a solution capable of predicting the likelihood of anemia through the analysis of Palpebral Conjunctiva images. This specific area of the eye offers valuable insights into an individual's health status, including potential signs of anemia. Medical research has established a strong connection between certain characteristics observed in Retinal Fundus images and the presence of anemia. By harnessing this connection, we aimed to develop a tool that could accurately detect anemia based on these visual cues, providing a non-invasive and efficient method for diagnosis.
To solve the above problems we narrowed down on an Artificial Intelligence-based approach with a robust software system stack to handle large-scale deployments. We envisioned devising a solution wherein the anemia could be predicted from the Retinal Fundus images as it is a good indicator of anemia according to existing medical research.
Building a good AI-based solution at a fundamental step requires the right data. So we started with the data collection process, fortunately, due to the client’s network, data collection was brisk. Since data was being collected with help of various devices, standardization of data was tricky since each device would have different hardware specifications. So rolling out a scalable solution wasn’t an easy task.
The Solution
Embarking on a journey of innovation, we developed a comprehensive solution for anemia detection, focusing on both model development and efficient deployment -
Deep Learning Model Development
We embarked on crafting a deep learning model specifically tailored for anemia prediction, leveraging pre-processed retinal fundus images as the primary input. Overcoming the challenge of selecting an appropriate deep learning architecture, our aim was to ensure the model's ability to generalize on data without falling prey to overfitting or underfitting. Our focus was on enabling the model to learn implicit, abstract, and relevant representations, rather than relying on manual feature engineering, thereby enhancing its predictive accuracy.
Prediction Capabilities
Utilizing advanced techniques, our solution implemented regression of haemoglobin count values within the model. Furthermore, we integrated downstream metadata-based logic to enable precise prediction of anemia severity across a spectrum of classifications, ranging from non-anemia to mild, moderate, and severe cases. This comprehensive approach ensured accurate and reliable predictions, facilitating timely intervention and treatment strategies.
Deployment as API Solution
Following model development, we encapsulated the entire solution into a user-friendly API format, addressing concerns related to scalability, portability, and the high maintenance requirements associated with invasive methods. Additionally, we embedded an extra layer of authentication interface within the software stack to bolster system security and safety, enhancing overall reliability.
Impact and Outcome
By crafting this solution, we've empowered smartphone users to effortlessly capture Palpebral Conjuctiva images, transmit them, and swiftly receive results—all without reliance on physical or infrastructure constraints. Remarkably, this process eliminates the need for medical representatives or manual intervention. Our solution is characterized by scalability, portability, reduced infrastructure requirements, cost-effectiveness, and user-friendliness. Notably, we've achieved accuracy levels higher than bulky and expensive state-of-the-art machinery. With this scalable solution, the goal to significantly impact the detection and treatment of anemia among the masses becomes a reality.
Accuracy in Anemia Detection
Model Inference Time
Read More Case Studies
Check out more Codemonk projects & success stories
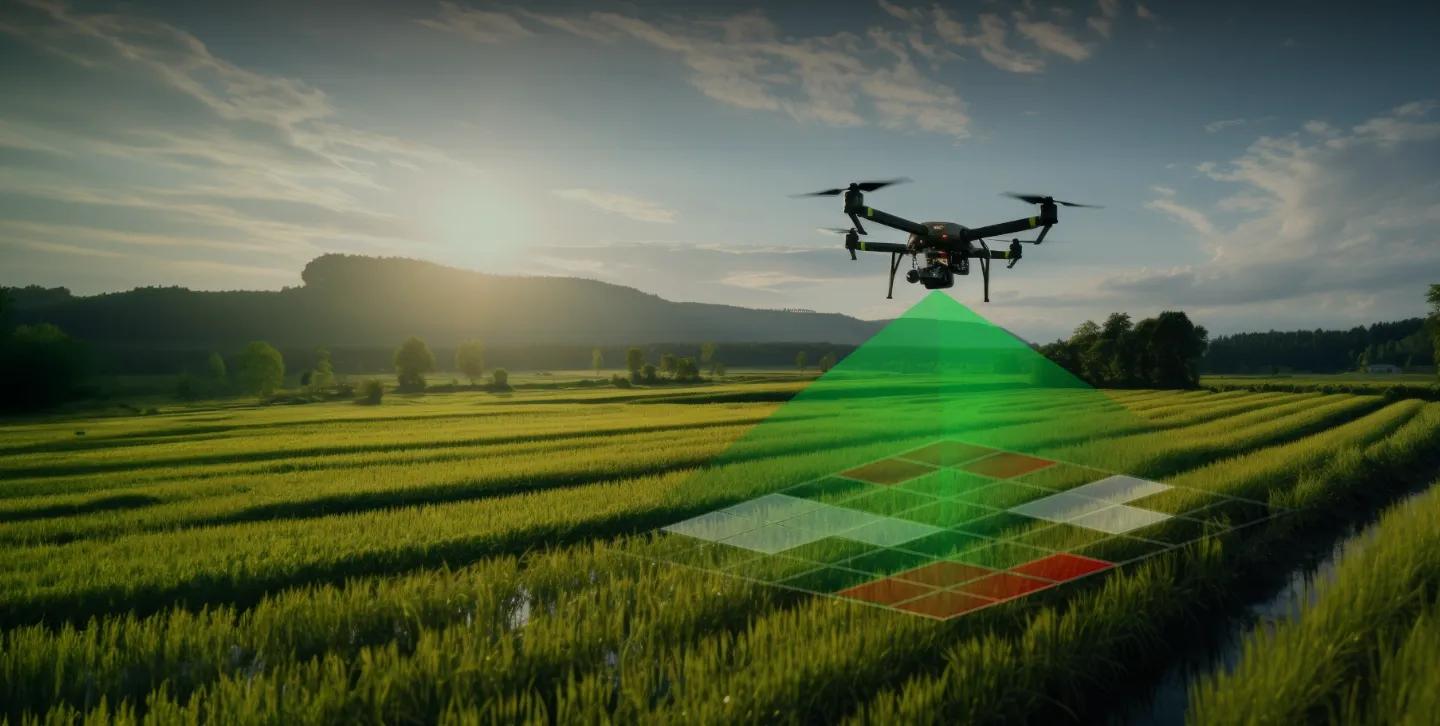
DRONETECH
Raw Pixels to Actionable Insights: Transforming Drone Data Analytics for Skylark Drones
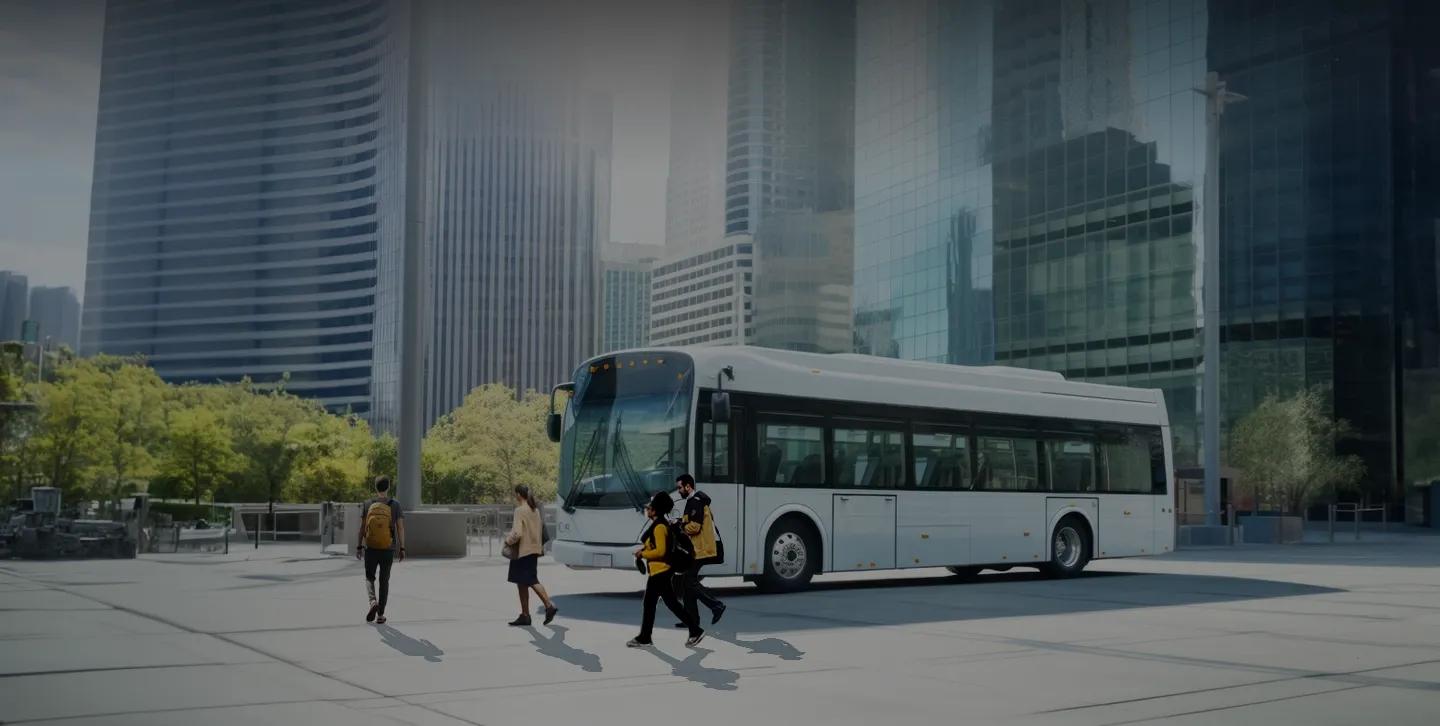
FMCG
Safeguarding Commuter Safety: Real-Time Bus Monitoring for Fortune 500 Company
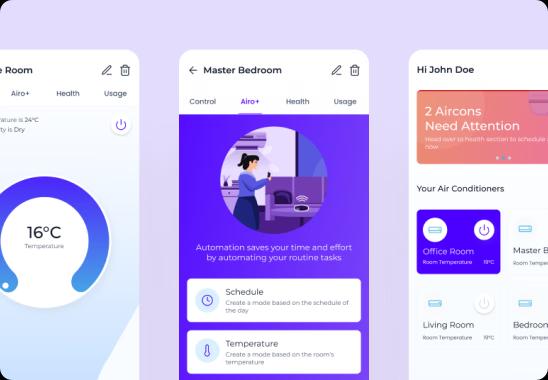
SUSTAINABILITY
Empowering Sustainable Cooling: Airo's Digital Ecosystem by Codemonk